Article updated on: 12 April 2024
Data-driven decision-making (DDDM for short) has become increasingly popular thanks to advances in AI and machine learning technologies. By pulling data from across the company, these solutions empower business leaders to make informed decisions in a fraction of the time needed.
This change gave rise to data-driven companies – businesses that value data as a strategic asset and use it to make decisions. According to Google, data-driven organizations are three times more likely to report significant improvement in decision-making.
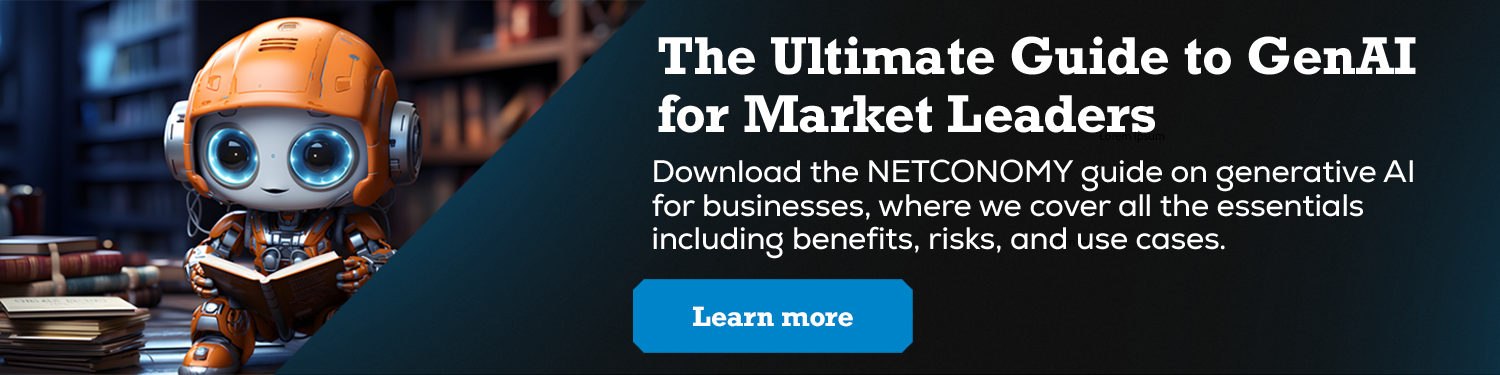
But humans have evolved to make decisions based on our gut instincts. That’s how we know not to go down a dark alley at night. Or not to eat that funny-smelling chicken that might have been sitting in your fridge for too long. The same goes for making business decisions – gut feeling is still the default for many of us.
For this reason, changing to data-based decisions requires a conscious effort, especially when the data reflects a reality different from what we expect or desire.
In this article, we’ll help you understand what data-driven decision-making is, why it’s useful, and how you can start benefiting from this approach.
Here’s what we’ll cover:
Let’s jump right in.
What is Data-Driven Decision-Making (DDDM)?
Data-driven decision-making (or data-based decision-making) involves using facts and relevant metrics to make decisions.
This type of decision-making can take many forms – from leveraging user testing when deciding on a new product feature to adding additional employee benefits based on the satisfaction survey results. A common thread is that you use data instead of instincts, subjective viewpoints, and gut feelings.
Empirical evidence gives you more confidence when facing tough choices that might impact your entire business. It allows you to turn raw data into actionable insights that you can use to address pain points, detect potential development opportunities, and stimulate profitability.
So, it’s not surprising that research shows that 65% of B2B sales organizations will transition to data-based decision-making by 2026.
Companies can leverage data-driven decisions regardless of size, industry, or products and services. However, implementing data-driven decision-making takes time and effort.
In the following section, we’ll explain why the benefits are more than worth it.
Benefits of Data-Driven Decision-Making
Adopting a more data-driven approach brings significant benefits for business leaders and, more importantly, the companies they are heading. As your teams adopt this new way of making decisions, you can expect to see:
- greater transparency and sense of ownership
- tighter collaboration
- better predictions of the future
- higher revenue and profitability
- and increased agility
Let’s look at each one in more detail.
Greater Transparency and Sense of Ownership
Data-driven decisions give your teams consistency and facts-based insights, empowering them to make better-informed choices.
All decisions are made based on data that is proactively shared across the company. As a result, everyone knows where the company is heading and what challenges it needs to overcome.
A typical result of this increased transparency is a greater sense of ownership. When people are aware of company goals and how their work directly impacts their overall success, they are more likely to take responsibility for their work. This ownership leads to increased motivation, productivity, and overall job satisfaction.
Tighter Collaboration Leading to Innovation and Knowledge Sharing
Data-driven decision-making nurtures better collaboration by giving multiple perspectives on a single issue. As different teams combine their unique viewpoints through the data, you have a chance to look at a problem from various angles before making a decision.
Tighter collaboration within and across teams often leads to more open and honest discussions. And these discussions are a great incubator for innovation.
Moreover, continuous data-sharing and closer teamwork help cultivate the transfer of knowledge and skills. That way, senior colleagues can share their experiences with new joiners while being exposed to fresh ideas and outside-of-the-box thinking.
More Accurate Predictions of Future Opportunities
The more market data you collect and analyze, the easier it becomes to spot emerging patterns. These new patterns allow you to identify gaps or potential market opportunities where others might see only challenges.
But the true magic happens when you combine generative AI and data analytics. By letting the model analyze vast amounts of past market data, you can train it to generate future scenarios that follow the same principles.
By tweaking certain variables in these future scenarios – for example, changes in customer sentiment or disruptions in global supply chains – you can plan your future steps more accurately and make the most of your connections, projects, and partnerships.
Higher Revenue and Profitability
When you know what your customers like or dislike, want, and need, it’s easier to decide what new products or services to answer with. That is one of the reasons data-driven organizations outperform their competition in profitability and productivity.
For example, a Forester Consulting research of 900 business leaders showed that data-driven companies are 162% more likely to surpass revenue objectives.
So it’s not surprising that retailers – who have to fight tooth and nail for every bump in their bottom line – have been aggressively adopting the data-driven approach. As many as 62% of surveyed retailers reported that data analytics provide an advantage over the competition. This shift is accompanied by widespread use of AI in retail to help find patterns in customer behavior that are not visible to human analysts.
Increased Agility
Once your data collection and analysis are up and running, you can have real-time data available whenever it’s needed. As a result, you and your teams can make decisions in seconds or minutes instead of hours.
Thanks to increased agility, you can quickly pivot and take advantage of new business opportunities or mitigate risks as they appear.
We experienced this benefit first-hand, working with one of our customers, an energy services brand recognized for its affordability. To help ensure their clients always get the best deal, we created an automated dashboard that tracks prices across the market, alerts their team in case prices are higher than the competition’s, and suggests a price change based on various other factors.
As a result, manual work is reduced to a minimum (before the change, teams had to check the market prices manually), while decisions are made based on the market situation as it develops.
Next, we’ll explore a couple of examples of world-renowned brands using this approach to further illustrate the benefits of data-based decisions.
Examples of Data-Driven Decision-Making
Google – Performance Reviews, Surveys, and Analytics
In 2002, Google eliminated all its project managers as part of an experiment to determine whether having managers was necessary. Not surprisingly, the answer was a strong yes.
However, Google also wanted to know why managers matter. So, they established a People Analytics Department to find that out and ensure that all future HR-related decisions are based on data.
Google had clear objectives (uncovering traits that make a great manager) and knew what information to look for. They reviewed performance reviews and employee surveys and interviewed people throughout the company.
Based on all that data, Google identified the eight factors that make a stellar manager. Then, to reinforce their data-driven approach, they introduced a biannual feedback survey to keep learning from their best people.
Netflix – Advanced Data and Analytics
Netflix has always been a leader when it comes to companies using data-driven decision-making. Their every decision – including choosing color palettes – is based on scientific insights and facts. This is why they have invested heavily in building teams of data engineers, analysts, and data visualizers.
Moreover, when choosing new shows, they rely on advanced content data and analytics tools to evaluate the performance of similar programs in the past. As a result, they minimize the risks of such high-stakes decisions.
These examples highlight the effort behind the data-driven approach and its benefits. Now, it’s time to show you how to implement data-based decision-making in your work and throughout your organization.
How to Make Data-Driven Decisions
The best way for leaders to promote data-driven decision-making is to lead by example. There are many different ways to make a decision based on data, but some of the common steps include:
- setting your objective
- choosing data sources
- organizing your data
- performing the analysis
- coming to a decision
Set Your Objectives
Formulate your goals and understand what you want to solve, identify, prevent, or achieve.
Whatever your goal is, make sure it’s clearly defined, measurable, and well-documented. Remember that analytics can only be effective if everyone is clear on what you’re trying to achieve and what you’ll do with the data.
For example, let’s say you want to increase your sales figures for this year.
Choose Your Data Sources
Choose the sources you’ll use to get your data. In our example, this would include sales figures for previous years. However, you should include data from marketing campaigns, social media or website analytics, and customer feedback surveys.
With so many sources, ensuring you have access to all the data relevant to your goals is crucial. Data silos can significantly damage an organization’s ability to see the big picture, resulting in bad decisions and even loss of money.
Finally, consider whether you can use the data for multiple projects. If yes, you need to plan on structuring the information to accommodate different scenarios.
Clean and Organize the Data
Data is only helpful if it’s accurate and organized in a way that makes sense to everyone. So, before you start analyzing, you need to eliminate or correct irrelevant, erroneous, or incomplete data.
This practice gave birth to a familiar trope: data scientists spend 80% of their time finding, cleaning, and organizing data while only 20% actually performing the analysis.
In our case, a customer data platform would be handy, as it would unify all customer data in one place and present it in a way that is easy for you to digest.
You can also have your data team develop visual tables and data dictionaries to organize insights and catalog variables. This step is necessary for an accurate and valuable conclusion.
Analyze the Data
Once the data has been collected and cleaned up, it’s time to run the analysis. Having the right skills and tools is crucial for this step. It’s great if your current team can do that in-house, but many companies need expert help with this part.
They’ll use statistical models (e.g., decision trees, clustering, time series, logistic regression) to analyze the data and decide how best to present the information.
Alternatively, you can turn to specialized AI solutions to do the analysis for you. However, it’s wise always to include a human in the process since the current versions of these models are prone to “hallucinations” and can provide false conclusions.
Turn Abstract Data into Actionable Insights
In the final step, it’s up to you to use the information to make a decision. It includes turning the insights you’ve generated into actionable steps to help accomplish the goal you’ve set initially.
In this step, you should be able to present the data and your findings so that everyone in your organization can understand how you arrived at your conclusion.
This understanding will ensure more confidence in your decision and promote data-based decision-making throughout your organization.
Supporting Your Decisions with the Right Technology
Choosing the right technology is crucial for effectively supporting data-driven decision-making within your organization.
Which solution you choose should depend on two factors.
- First, you need to ensure that the technology fits your needs and the goals you’re trying to achieve, not the other way around.
- Second, look for solutions that bring together diverse data sources, but also connect with one other. It doesn’t matter how much data you have if you can’t analyze it.
Once you have these two figured out, you’ll have a rough idea of what kind of solution you need. If you are primarily interested in customer data, a customer data platform would be a great solution. On the other hand, if you want to unify data from across your organization, a data warehouse would be a better bet.
When working with customers, we always start by discussing their business goals and assessing the status quo of their technical environment. This way, our solutions consider both the technological and business perspectives, ensuring your future success.
Frequently Asked Questions (FAQ) About DDDM
What are the main challenges of data-driven decision-making, and how can I overcome them?
The two critical challenges of data-driven decision-making are data overload and low-quality data. Solving data overload requires prioritizing critical data that directly influences your decisions. On the other hand, addressing data quality and bias involves rigorous governance, quality control, and ongoing education on data literacy.
What specific metrics or KPIs should I focus on when implementing data-driven decision-making?
The specific metrics you’ll focus on depend mainly on your goals, industry, and the aspect of the business you want to improve. Standard metrics you could pick include customer satisfaction scores, employee engagement levels, operational efficiency measures, and financial KPIs such as revenue, profitability, or cost savings. Be prepared to regularly review and adjust these metrics as your business and the environment evolve.
What ethical and regulatory considerations should I pay attention to regarding data-driven decision-making?
Ethical considerations are crucial in data-driven decision-making, particularly regarding data privacy, consent, and bias. The best ways to protect your brand from harm and avoid substantial regulatory fines are to collect and use data in compliance with relevant laws, obtain consent where necessary, and protect your customers’ personal data.
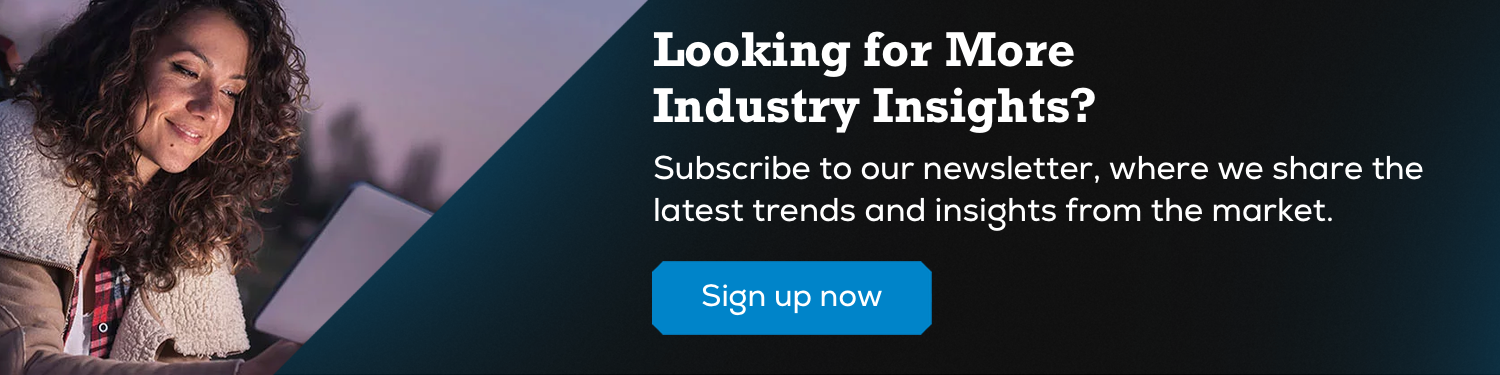