Generative AI is rapidly becoming a global phenomenon and its business use cases – from generating content in the blink of an eye to powering virtual customer service agents – are set to revolutionize the way we do business.
And even though there is much discussion about the influence of this revolutionary technology on wider society, its negative implications for companies are often overlooked.
In this article, NETCONOMY experts on generative AI – Boban (Development Lead) and Manuela (Experience Management Consulting Lead) – explore the risks of generative AI in detail and share their first-hand experience on how to manage them.
The five key risks of generative AI for businesses are:
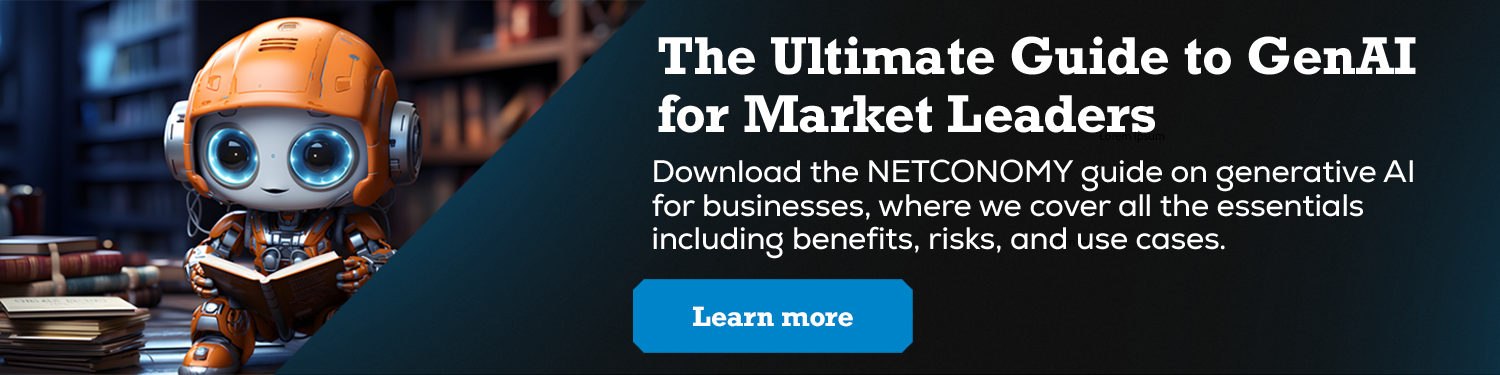
Accuracy of Generative AI Output
The biggest concern of generative AI for data-driven companies is the accuracy of its output, particularly the risk of hallucinations. These hallucinations are actually cases when AI generates responses which are incorrect or irrelevant. And since these responses are worded confidently and seem to be underpinned by sound logic, they often appear true.
Inaccurate responses pose significant risks to companies, as they can lead to misinformation and confusion. And if you’re in a habit of making decisions based on data, this ultimately leads to damaging your reputation and customer trust. To make matters worse, large language models rarely produce the same output twice, making fact-checking difficult.
Grounding and Testing
However, companies can take steps to reduce the risk of hallucination through the process of grounding. Grounding involves anchoring the AI system to reliable data or a source of truth.
For example, the RAG (Retrieval-augmented generation) approach uses grounding by retrieving relevant documents (for example, your product documentation) and generating responses based on them.
Another way to test your model is to compare the output to a dataset you consider the truth. If you’re setting up a Q&A chatbot, the truth would be the replies from your customer service agents.
You then ask the system to answer the same questions and compare the two data sets. Based on the results, you can see how precise the model is and the potential gaps you need to address.
Educating Customers and Teams
But improving generative AI goes beyond just technological advancements; companies must also invest in educating their staff and customers.
Many teams hold a misconception that data quality doesn’t matter with generative AI because of its ability to read unstructured data. However, the saying garbage in, garbage out still applies, so it’s crucial to ensure reliable data feeds into the system.
On the other hand, users need to be aware of the capabilities and limitations of generative AI and use it with appropriate assumptions and considerations.
User Feedback
Lastly, continuous user feedback is vital in improving generative AI models. In our projects, we make it easy for users to provide feedback on the answers they get by enabling them to like or dislike AI responses.
For greater clarity, you can ask them why a particular answer wasn’t good (for example, if it wasn’t relevant) and even leave an option to comment for additional context. This feedback will help identify gaps within the model and develop strategies to address them.
Risk of Generative AI Bias
Most generative AI models are trained on data scraped from the internet. And while it might be tempting to assume that the internet is a good representation of the world, that’s far from the truth.
Access to the internet is not equal, and many parts of society are not heard or are marginalized.
As a result, our broader societal biases can seep into generative AI models that can then:
- amplify stereotypes (for example, through the content they generate)
- make discriminatory decisions
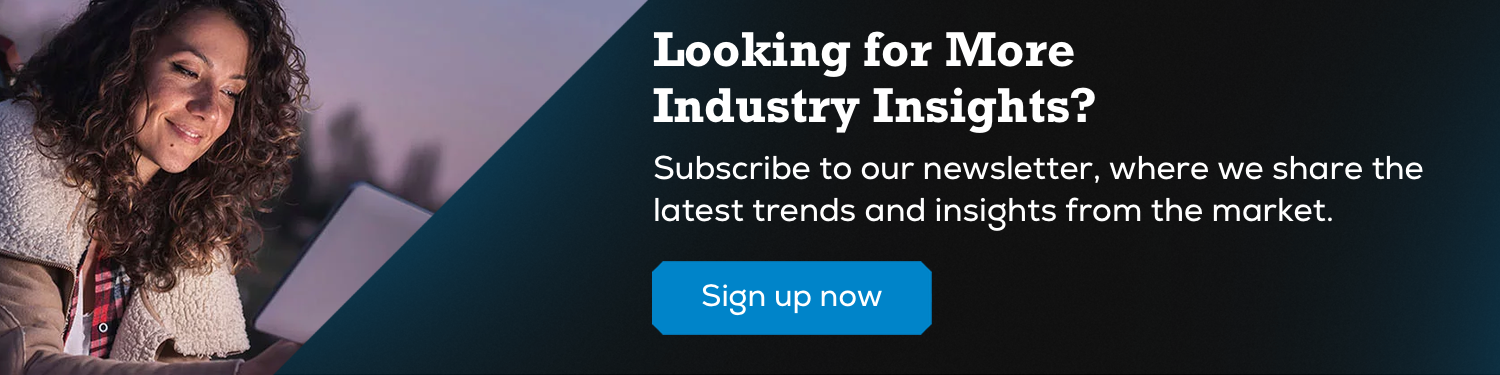
Companies should prioritize using high-quality data sources (such as first-party data) as the first step to mitigating this issue. In addition, they should actively work to identify and eliminate bias in their datasets. This can include strategies such as diversifying the training data to be more inclusive.
Another approach is to have humans guide the AI models – a practice known as Reinforcement Learning from Human Feedback. While not explicitly designed to combat bias, this practice can be highly beneficial if companies have the capacity and expertise to implement it.
Transparency is also crucial if you want your generative AI model to be free of bias. Frequently, AI models operate as a black box leaving users unaware of the process behind a specific outcome.
The rise of Explainable AI (XAI) aims to address this by measuring a model’s interpretability and explainability. This transparency enables users and experts to understand the ‘How’ and the ‘Why’ behind a specific answer and provide feedback.
Moreover, there is a growing societal demand for ethical AI development that considers the perspectives of marginalized groups. Initiatives such as the OECD’s AI Policy Observatory and the efforts of various freelancing networks highlight this commitment.
Data Privacy and Security Risks of Generative AI
Generative AI poses two significant risks for companies regarding data privacy and security:
- External – where customers share confidential information that is stored and (potentially) misused or revealed.
- Internal – where employees may inadvertently give away confidential company information through prompts.
If your company uses customer data to train models, you must anonymize this data first. There are several methods to choose from, such as masking (replacing this information with equivalent random characters or fake data) or tokenizing (swapping information with a one-of-a-kind symbol).
At NETCONOMY, we use Google’s Data Loss Prevention solution to ensure all personally identifiable information (PII) is automatically identified and anonymized before being fed into our models.
In the case of AI chatbots or shopping assistants, there is an additional layer of risk as these solutions often handle critical information such as credit card details.
In these situations, it’s crucial to keep this information only as long as necessary (e.g., until they are forwarded to the payment provider) and ensure it’s then deleted from your logs to prevent unauthorized access.
Internally, businesses need to invest in training their workforce (something we already mentioned) and also provide vetted and compliant generative AI tools.
Gen AI solutions are not going away, and not using them would only keep you from achieving great benefits. The question you need to ask instead is – how can we make these tools available internally in a safe way?
Finally, the complexity of the digital landscape further complicates these data security issues. For instance, let’s say you want to connect your customer data solution to a generative AI solution from another vendor.
In this case, all previous advice about anonymization applies. But as you now have two solutions working together, you need to ensure these are followed twice.
The situation can become even more complex when you consider who has access to both solutions, whether both are adequately maintained and so on.
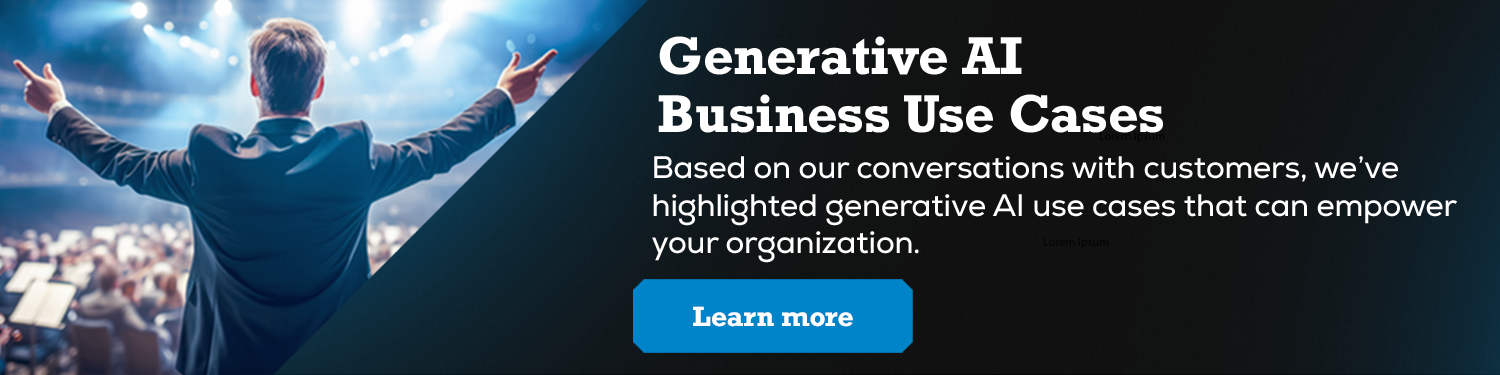
Ethical Risks of Generative AI
The most significant ethical challenges of generative AI for society at large are connected to deepfakes that are almost indistinguishable from reality. However, the ethical risks for businesses primarily revolve around the potential for job cuts, a common outcome of technological revolutions.
The term “computer” was once used to refer to people who performed long, complex mathematical calculations supporting scientific or business work. However, this significantly changed with the introduction of digital computers.
However, the current revolution with generative AI has the potential to reshape our work environment within a few short years rather than decades. This condensed timeline puts additional pressure on businesses to ensure they do not neglect their broader societal responsibilities in pursuit of profit.
Consider the example of software developers. With the introduction of GitHub Copilot, companies may be tempted to believe they no longer need junior developers.
However, these companies overlook the fact that years of hands-on experience and accumulated knowledge are crucial for individuals to progress into more senior roles, such as software architects.
By eliminating these junior roles, businesses risk losing this vital know-how and potentially jeopardizing their long-term success.
Menial jobs are particularly susceptible to the impact of AI, and companies must proactively plan for the future by facilitating the transition of these workers into roles that align with their skills and experiences or by providing opportunities for upskilling into new positions.
Lastly, we must remember that this technology will create new jobs requiring new skills and work methods we cannot even imagine – much like how the past ‘human computers’ could never have envisioned the work of modern software developers before the arrival of the first digital computer.
Intellectual Property and Legal Risks of Generative AI
We know most gen AI models are trained using an array of internet content. So it’s only logical that much of that content will be subjected to intellectual property and copyright protection.
This became painfully obvious when Getty sued Stability AI for allegedly using over 12 million of their photos to train AI models. Something that was easily provable since some images produced by Stability’s model also included the Getty watermark.
This example clearly illustrates why it’s risky for businesses to use content generated from copyrighted material. And even though both OpenAI and Google now offer to protect their customers from copyright challenges, this doesn’t eliminate the problem.
Even when using freely available data from the internet, broader questions arise.
Consider a scenario where a business scrapes data from a competitor’s website and uses an AI model to automatically undercut their prices in real time.
Even though this information is not copyright protected, the question of whether we should endorse this kind of business behavior comes up.
You might say this can also be done manually by checking a competitor’s website and updating your own prices. But the scale and speed at which generative AI can achieve this introduces broader societal implications.
The industry is currently similar to a playground, full of grey areas. And many businesses are left wondering whether their use case might violate future regulations.
So having the right partner to help navigate and flag potential challenges and risks that might surface downstream becomes paramount.
Final Thoughts on the Risks of Generative AI for Business
In conclusion, it is essential to recognize that every new technology, including generative AI, comes with inherent risks. These risks, which include data security, ethical concerns, and intellectual property issues, pose significant challenges.
However, this doesn’t mean companies should avoid using generative AI models and their many benefits – quite the opposite.
They need to familiarize themselves with its capabilities, limitations, and underlying mechanisms. By doing so, they can better manage the risks and ensure their use of generative AI aligns with societal and legal expectations.
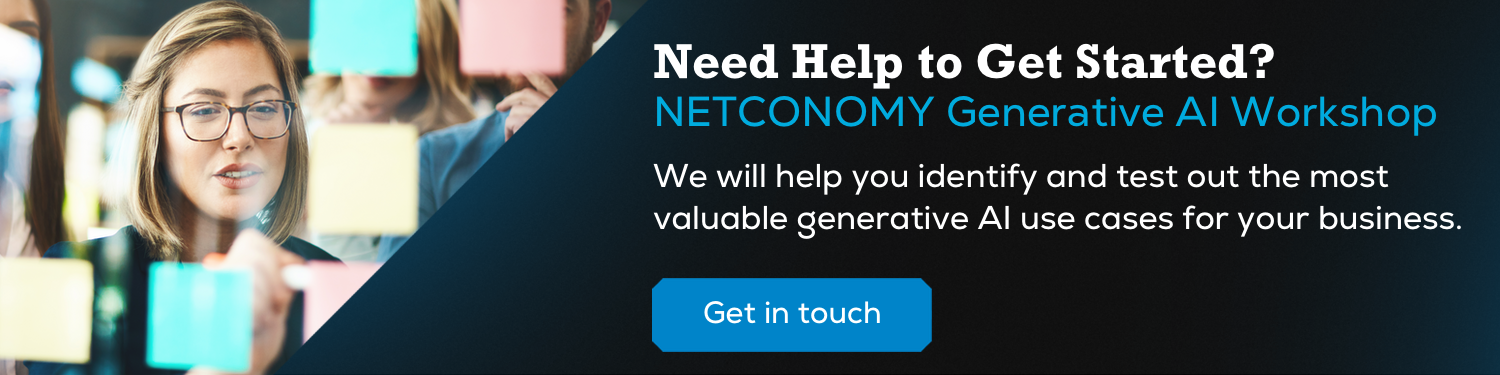