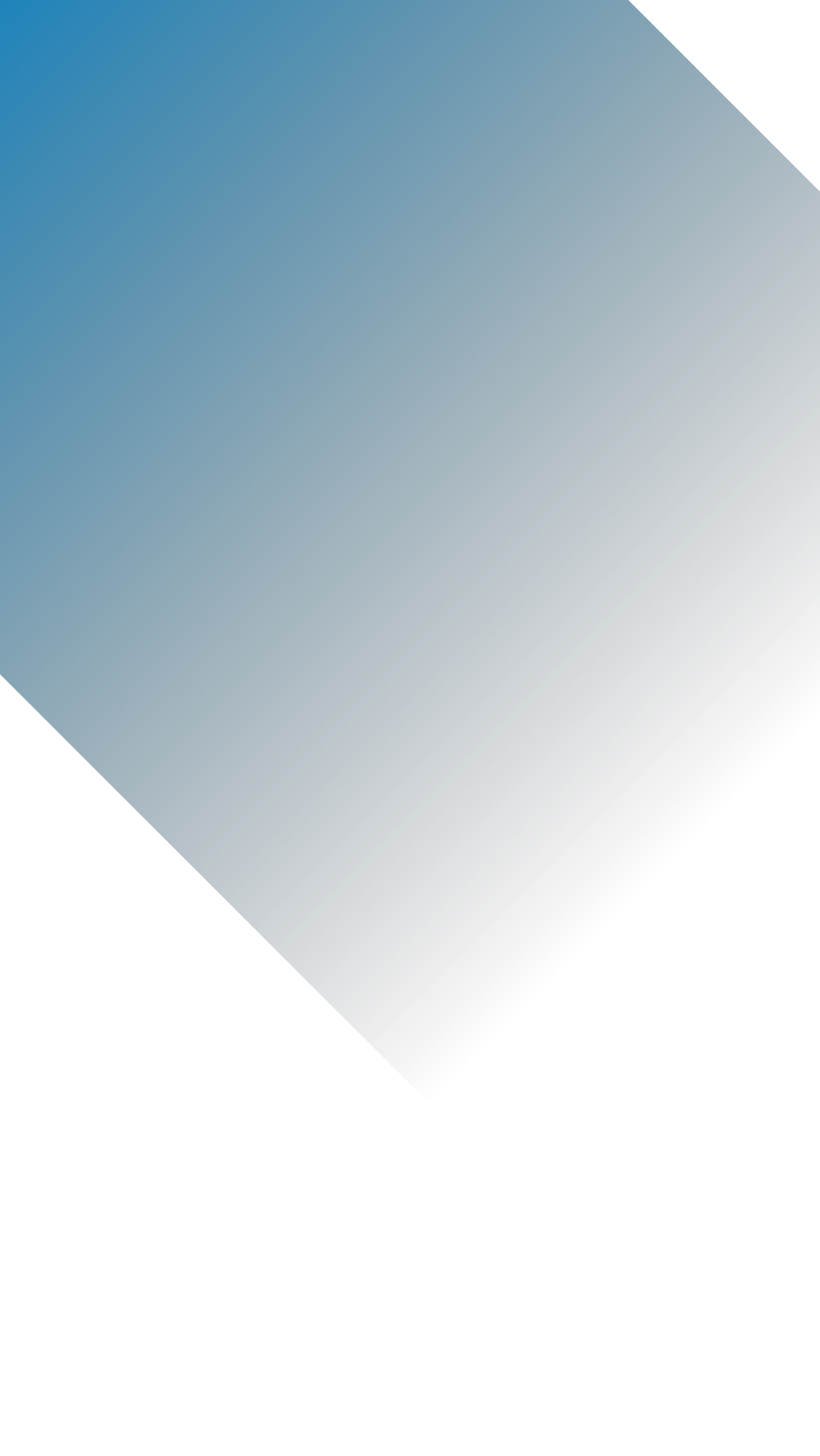
Big Data vs. Smart Data: Is More Always Better?
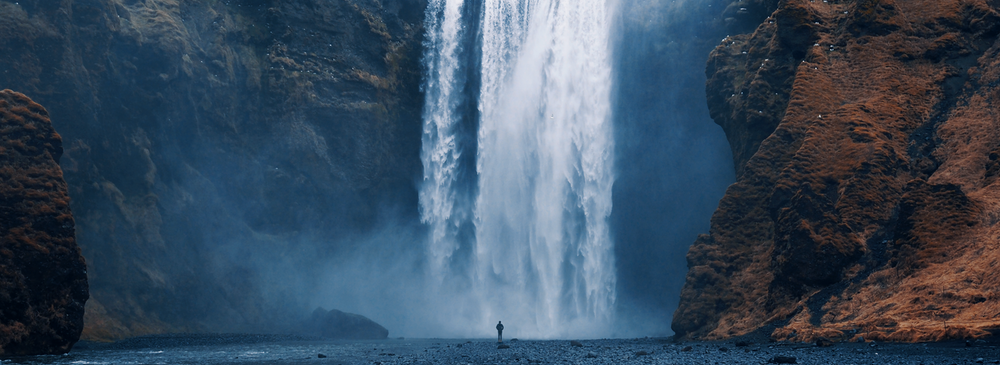
Article updated on: 29 February 2024
Imagine standing near a powerful waterfall, deafened by the noise of the surging water. The feeling is overwhelming, and you struggle to take in the details – the rock covered by moss, a bird flying overhead, a small fish jumping in the pond. There is simply too much, and these small parts of the picture become invisible.
In today’s world, big data is the waterfall. Business leaders are repeatedly told that data is the new gold and that they can’t survive without it – giving rise to data-driven companies. But as businesses struggle to collect and analyze everything, they miss important details that might have helped them take the lead. And here is where smart data comes into play.
Here’s what we’ll talk about:
- What is big data
- What is smart data
- Differences between big data and smart data
- Extracting smart data from big data
- Using smart data with artificial intelligence solutions
What Is Big Data?
Big data represents colossal amounts of structured and unstructured data that organizations and businesses collect daily. Big data can come from any number of sources – from social media and Google search to data gathered by IoT sensors on the product line.
Whatever the source, the goal of collecting big data is getting actionable insights and information to solve business challenges, improve customer experience and make operations more effective.
What separates big data from other data sets is that due to its size, it is difficult to process using traditional data processing techniques.
We can better define big data by using the following four dimensions – the 4 Vs of big data:
- Volume: the amount of (structured and unstructured) data generated and stored.
- Variety: the different types of available data such as text, images, videos, etc.
- Veracity: the credibility and quality of data, including accuracy, consistency, and completeness.
- Velocity: the speed at which a business generates and processes the data.
So, the four Vs of big data show that it is large, fast-moving, diverse, and might be of lower quality. These characteristics make it challenging to store, manage, and analyze big data in order to get actionable insights.
Now that we know what big data is, let’s look at smart data and how it mitigates some of these challenges.
What Is Smart Data?
Smart data represents smaller data sets that provide meaningful, valuable, and actionable information. Smart data is extracted from big data by filtering, cleaning, and organizing data at the collection point, making it easier to analyze and act on.
Advanced analytics and machine learning algorithms can extract smart data from structured and unstructured texts, documents, attachments, emails, etc., to ensure it makes sense and can produce valuable insights.
An important thing to keep in mind is the purpose of collecting the data. This goal will later determine which dimensions of the data set are important, how it will be filtered, etc.
To illustrate this through an example, let’s say we have a retail store that collects data on past purchases, the item price, and customer demographics.
If our goal is to increase sales, we can analyze the data set to find which item is sold the most to which demographic. Then we can use this data to stock up and invest in marketing for this specific group of customers.
On the other hand, if we want to optimize our prices, we can analyze the data to see how price changes impact the demand for different items. Based on this information, we can then set prices at levels that maximize profit.
As you can see, the same smart data set can provide different insights and support different business goals, depending on which dimensions are analyzed.
When we look at smart data in the context of the 4 Vs of big data mentioned above, we can say that smart data focuses on veracity and adds the fifth V – value. This is because it’s more reliable and up-to-date due to being current, complete, consolidated, and cleaned.
Since we now understand the basics of both big data and smart data, we can cover the main differences between them.
Read more: The Ultimate Guide to Generative AI for Market Leaders
Differences Between Big Data and Smart Data
Big data was a buzzword of the previous decade. However, as the amount of data businesses generate increases exponentially, companies are struggling to get the ROI from their investments in big data that they had anticipated. That’s why companies are now using advanced AI and machine learning algorithms to extract smart data out of big data, removing many of its shortcomings.
In the context of the four Vs of big data, the most significant difference is that big data focuses on volume, while smart data is all about quality – and all other differences stem from this simple fact.
Smart Data Is Better Targeted
Many companies use big data to stay ahead of their competitors. However, it’s difficult to ensure big data aligns with business priorities and pain points, decreasing its value. On the other hand, smart data is more targeted and precise, helping companies use it in a practical and valuable way.
Smart Data Is More Accurate and of Greater Quality
Smart data is screened and filtered, allowing better decision-making and higher efficiency. According to a Deloitte survey, big data is often inaccurate, and more than two-thirds of respondents said that less than half of the third-party data about them was correct.
Smart Data Provides Actionable Insights in Real-Time
Smart data is readable and helpful from the get-go, giving actionable insights as the data is being collected. On the flip side, big data requires processing to become usable and can create data silos.
Smart Data Ensures a Higher Customization Level
Big data doesn’t provide context and isn’t tailored to specific business requirements and standards. However, smart data provides precise information that fits a company’s unique industry context and needs, allowing better outcomes and solutions.
Smart Data Can Be Beneficial in Machine Learning
In machine learning, more data is often not better. It turns out that some machine learning algorithms hugely benefit from being trained with less but more specific data.
From Big Data to Smart Data
Advances in Artificial Intelligence (AI), machine learning (ML), and data analytics drive the rise of smart data by processing big data from multiple sources and transforming it into actionable insights.
Machine learning methods allow companies to automatically process and filter data without the need for a data scientist to do manual work. This greatly boost the speed and accuracy of big data processing.
However, the fundamental shift from big data to smart data starts with the question, ‘why am I collecting this data.’ That shapes how we collect, process, save, and finally use it in decision-making.
After all, gathering and analyzing data is only meaningful when companies can use it to address their unique problems. Because of that, businesses are shifting from just amassing vast amounts of uncontextualized data to also processing the information, which makes it easier to identify patterns, trends, and anomalies.
Smart Data and Artificial Intelligence Solutions
According to a Gartner report, more than 85% of AI projects fail. Data quality is among the most common reasons for this failure since many companies feed AI systems data without assessing, refining, and modifying it.
Implementing AI and machine learning projects based on smart data is the most efficient and effective way. This approach allows companies to develop quality projects that align with their business needs, context, and objectives.
By eliminating the noise of big data’s volume and focusing on quality information – companies can use and interpret data more accurately, driving the success of their programs.
In general, smart data helps companies enhance product quality, manage operational risks, establish predictive models, understand their customer behavior better, and lower sales-related expenses. The world can benefit from understanding the difference between big data vs. smart data and acquiring a smart data mindset, as that helps AI have a more meaningful impact on society and the economy.
Frequently Asked Questions
- What specific challenges do companies face when transitioning from big data to smart data?
One challenge for companies is handling large data volumes. With the amount of data growing exponentially, finding valuable insights is like searching for a needle in a haystack. Sophisticated algorithms and analytics tools are essential to discover patterns in vast datasets.
The shift also requires a cultural change towards quality over quantity. This involves retraining staff, adopting new technology, and prioritizing actionable insights.
Finally, data quality and integration pose significant hurdles as organizations gather data from various sources. Ensuring consistency, accuracy, and reliability becomes complex, demanding advanced data management solutions and a strong understanding of data science.
- How do companies ensure the privacy and security of smart data?
To protect smart data, companies use strong data governance rules, including clear policies on data access, use, and sharing to comply with GDPR and CCPA.
However, encryption is key in safeguarding data at rest and in transit, making it unreadable to unauthorized users and reducing breach risks.
Firms also turn to blockchain to boost security, as its decentralized nature thwarts hackers and adds a layer of protection for sensitive info.
- Can smart data be applied in all industries, and are there examples of industries where smart data has had a particularly transformative impact?
Smart data can be used across all industries, revolutionizing practices and fostering innovation. However, the use of smart data has a particularly transformative effect in the retail, healthcare, and financial industries.
In retail, personalized customer experiences are enhanced through data analysis of purchasing behaviors, leading to tailored recommendations.
In healthcare, predictive analytics improve patient care by forecasting outbreaks, identifying at-risk individuals, and customizing treatment plans.
The financial sector benefits from smart data for fraud detection and risk management.
Authors and Contributors
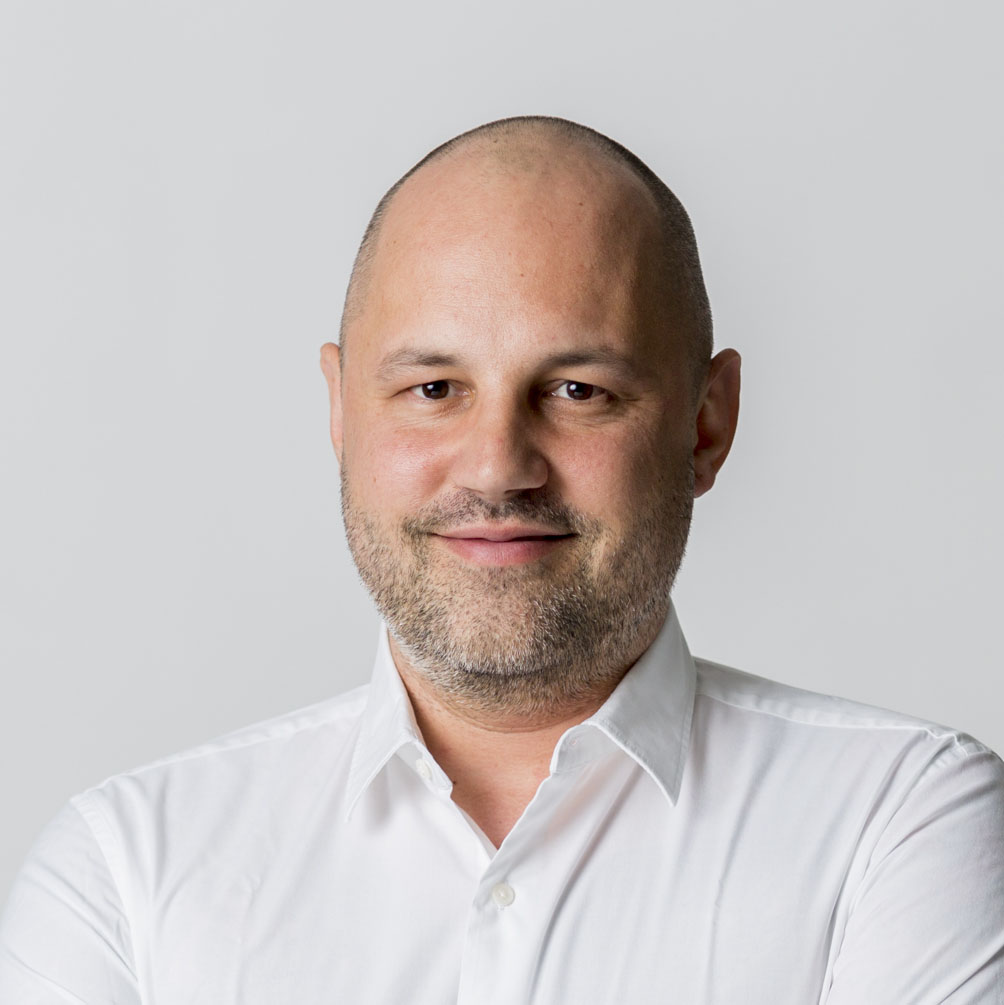
Martin Bachler | Chief Technical Officer, NETCONOMY
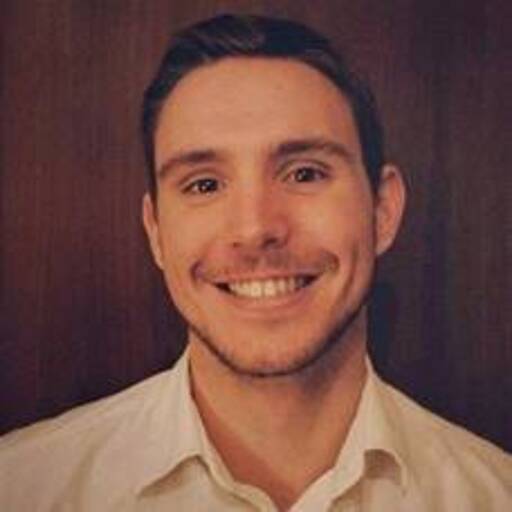