Machine learning (ML) and artificial intelligence (AI) technologies have been a part of our lives for a long time. We are now used to asking Siri to check the weather or having Alexa automatically reorder our groceries.
However, since the launch of ChatGPT, it has become clear just how much artificial intelligence technologies are involved in our lives – and this adoption trend is far from over. According to Fortune Business Insights, the machine learning market was valued at USD 15.44 billion in 2021 and is expected to skyrocket to USD 210 billion by 2029.
This exponential growth is driven by numerous applications of AI in different industries – from quicker diagnosis of diseases in healthcare to self-driving cars in the automotive sector.
In digital commerce, understanding customer behavior is crucial to achieving business success. Machine learning has become a game-changer here, enabling companies to analyze vast amounts of data and gain valuable insights about the customer journey. Global enterprises like Amazon, Alibaba, and eBay already use advanced algorithms to personalize their offering, increase sales, and ultimately provide customers with a more tailored and efficient experience. However, the success of these models highly depends on the quality and accuracy of the data used to train them. Machine learning engineers and data scientists play a critical role here, as they are responsible for designing, building, and maintaining the data infrastructure that powers these models.
In this blog, we will explore how our Machine Learning team works, how we use AI at NETCONOMY, and how our engineers make it all possible. (If you want to learn about machine learning or artificial intelligence but are not yet familiar with these topics, our blog briefly explains both and their differences.)
Machine Learning at NETCONOMY
We are actively working on becoming a more data-driven company and use AI/ML technologies in various areas, such as internal financial and resource forecasting. This way, we can create more accurate future scenarios and significantly reduce our manual efforts.
For our clients, we build and operate machine learning models and data management systems to:
- enhance customer experience on their digital platforms
- improve their businesses through automated processes
- extract actionable insights from the available data
Following are two use cases our Machine Learning team has been working on for our clients:
Product Visual Similarity Search
Imagine seeing a photo of a product online, and immediately deciding you have to buy it. The next step is usually visiting an online store and trying to find it by typing the description in the search function. However, we all know that these often spit out a large number of similar products you then have to dig through manually. To help with this, we developed a visual similarity search system that uses cloud image ML services and open-source models. As a result, customers can quickly and easily find similar items in the online shop by simply uploading a photo of a desired product. In addition, the system can analyze the uploaded data and provide personalized interactions. For example, if someone uploads a photo from a news article about a football club, the system tells them “It looks like you’re a football fan!” – and shows them the fan merchandise from the club seen in the picture.
Product Data Analysis and Product Recommendations
When adding new products to their online store, suppliers often struggle with their internal taxonomy and don’t know how to properly tag their products. As a result, customers can’t see all the relevant products when searching. To solve this, our team developed a solution that automatically analyzes products and enriches their data based on available media. The system extracts information from descriptions, images, videos, or external ratings and creates structured attributes – e.g., “shoes” – for each product. This way, suppliers can easily add new or update existing products instead of uploading the information manually, which takes more time and risks human error. Moreover, the system automatically creates clusters of products based on the processed information. This is then used to show customers product recommendations or similar products to the one they are looking at.
Find out more about one of our machine learning projects, which won an Innovation Award from the SAP AppHaus Network.
Our Team
As machine learning continues playing a critical role in various industries, the demand for a skilled workforce in this area will continue to grow. However, there is some uncertainty about the different roles, their names, and exact responsibilities.
Data Scientists are responsible for transforming raw data into actionable insights and building the data storage and processing infrastructure. Machine Learning Engineers then use this data to build models that can predict future events or outcomes. Finally, MLOps Engineer is a newer role that takes over the deployment and continuous maintenance of the developed models.
AT NETCONOMY, we combine those three roles into a hybrid role. This means that all our machine learning team members take on tasks in different areas – depending on their skills, talents, and interests. This approach ensures that our people can develop in the area that is most beneficial for them while contributing to a positive workflow within the team.
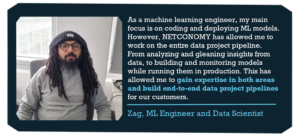
Aid is one of our ML engineers and leads the Machine Learning team. We caught up for a coffee and asked him to share some insights about what it’s like to work in their team, and here’s what he told us:
How do you work as a team?
Our team includes four machine learning engineers and a dedicated product owner who helps us stay organized. To ensure effective project management, we assign at least two machine learning engineers to each project based on its scope. We are also a distributed team, with two colleagues based in Germany, two in Austria, and one in Serbia. This means clear communication is crucial, so we try to make the most of our daily meetings. There, we discuss our progress and brainstorm solutions for any challenges we encounter. Good communication is definitely one of our team’s strengths, next to our willingness to share knowledge, which allows us to learn and grow together every day.
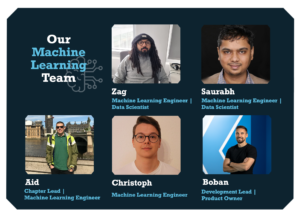
Which machine learning models do you use?
The model highly depends on the use case or project – and we had a lot of trial and error on the way. We’ve learned that XGBoost models work best for tabular problems that need to be trained on a vast amount of data. For classification problems – like our image recognition use case – we decided to train deep Convolutional Neural Networks because that proved most effective. Sometimes we go with a pre-trained model and finetune it according to the project requirements.
When choosing a model, we are free to go with whatever makes the most sense. First, we do a lot of research to find different ways to solve a certain problem. It can also happen that ideas from the research phase don’t work out in practice, so we go back and try another solution.
What was one highlight that you had as a team?
Undoubtedly, one of the most significant highlights was the first time we successfully implemented an ML model for one of our clients. It was special because the deployed model required a proper monitoring solution in production. While cloud providers simplify the model monitoring to some extent, our challenge was that we deployed the model in the client’s infrastructure, where we could not use the out-of-the-box cloud solutions. So we needed to build a custom monitoring solution.
What was one learning that you had as a team?
As a team, we’ve learned that holding regular meetings with our clients throughout the project phase is crucial. This allows us to communicate our approach and results effectively to ensure we are on the same page. When working with customers who are new to the machine learning space, it’s crucial to gain their understanding and align expectations with our own to avoid any potential discrepancies. We also learned that while machine learning projects share similarities with standard software engineering projects, one key distinction is that deploying a model into production requires considerable time and effort. This is because most problems often arise after the model has been deployed. The preparation of our ML infrastructure, including pipelines and model monitoring, and our ability to detect and address these issues were a particular learning experience.
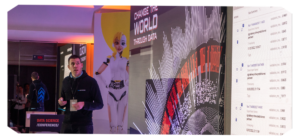
Aid taking the stage at the Data Science Conference 2022
Christoph is the youngest member of our machine learning team – he works part-time while studying computer science. He’s a great example of how we help students working at NETCONOMY balance their working hours depending on their university workload.
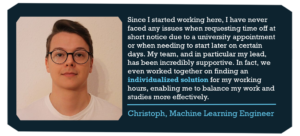
Of course, we want to offer all our employees the greatest possible flexibility. That is why our flexible working models give everyone the freedom to find their balance according to their unique situation.
Fostering the next generation of (Machine Learning) pioneers
While we give our people room to grow and motivate them to take charge of their development, we also want to help young people start their careers in the tech industry. That’s why we organize our annual Talent Lab for recent IT graduates, providing them with mentoring and real-life experience in software development.
However, we also want to engage with young people at an earlier stage, such as high school, and help them gain experience and know-how in different areas related to IT.
Therefore, our colleague Lukas, software architect and development lead, worked out an assignment around machine learning for the students of the HTL Mößingerstraße as part of their final thesis. There, the students were asked to develop an app that allows users to upload photos of outfits and quickly and easily find similar products to those shown in the image. The app analyzes which items of clothing can be seen in the photo and searches for the extracted terms in our store.
The project is soon to be done – and we can’t wait to see their innovative ideas brought to life!